Self-consistency cycle
The following section discusses the minimization algorithms implemented in VASP. We generally have one outer loop in which the charge density is optimized, and one inner loop in which the wavefunctions are optimized.
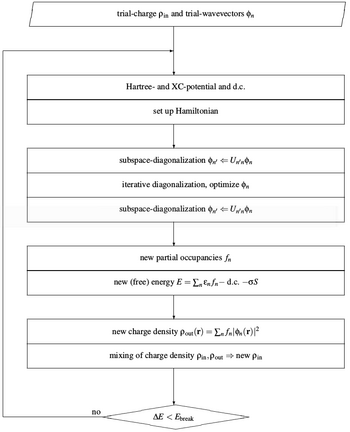
Most of the algorithms implemented in VASP use an iterative matrix-diagonalization scheme, where the used algorithms are based on the conjugate gradient scheme [1][2], block Davidson scheme [3][4], or a residual minimization scheme -- direct inversion in the iterative subspace (RMM-DIIS) [5][6]. For the mixing of the charge density an efficient Broyden/Pulay mixing scheme [6][7][8] is used. Fig. 1 shows a typical flow-chart of VASP. Input charge density and wavefunctions are independent quantities (at start-up these quantities are set according to INIWAV and ICHARG). Within each selfconsistency loop, the charge density is used to set up the Hamiltonian, then the wavefunctions are optimized iteratively so that they get closer to the exact wavefunctions of this Hamiltonian. From the optimized wavefunctions, a new charge density is calculated, which is then mixed with the old input-charge density.
The conjugate gradient and the residual minimization scheme do not recalculate
the exact Kohn-Sham eigenfunctions but an arbitrary linear combination
of the NBANDS lowest eigenfunctions. Therefore it is in addition necessary to
diagonalize the Hamiltonian in the subspace spanned by the
trial-wavefunctions, and to transform the wavefunctions accordingly
(i.e. perform a unitary transformation of the wavefunctions,
so that the Hamiltonian is diagonal in the subspace spanned by transformed wavefunctions).
This step is usually called sub-space diagonalization (although a more
appropriate name would be, using the Rayleigh-Ritz variational scheme in
a subspace spanned by the wavefunctions):
The sub-space diagonalization can be performed before or after the conjugate gradient or residual minimization scheme. Tests we have done indicate that the first choice is preferable during self-consistent calculations.
In general, all iterative algorithms work very similarly. The core quantity is the residual vector
This residual vector is added to the wavefunction , the algorithms differ in the way this is exactly done.
References
- ↑ M. P. Teter, M. C. Payne, and D. C. Allan, Phys. Rev. B 40, 12255 (1989).
- ↑ D. M. Bylander, L. Kleinman, and S. Lee, Phys Rev. B 42, 1394 (1990).
- ↑ R. Davidson, Methods in Computational Molecular Physics edited by G.H.F. Diercksen, and S. Wilson Vol. 113 NATO Advanced Study Institute, Series C (Plenum, New York, 1983), p. 95.
- ↑ B. Liu, in Report on Workshop "Numerical Algorithms in Chemistry: Algebraic Methods" edited by C. Moler and I. Shavitt, (Lawrence Berkley Lab. Univ. of California), (1978), p.49.
- ↑ D. M. Wood and A. Zunger, J. Phys. A 18, 1343 (1985).
- ↑ a b P. Pulay, Chem. Phys. Lett. 73, 393 (1980).
- ↑ S. Blügel, PhD Thesis, RWTH Aachen (1988).
- ↑ D. D. Johnson, Phys. Rev. B 38, 12807 (1988)